AI-Enabled Teaching and Learning in Adult Education

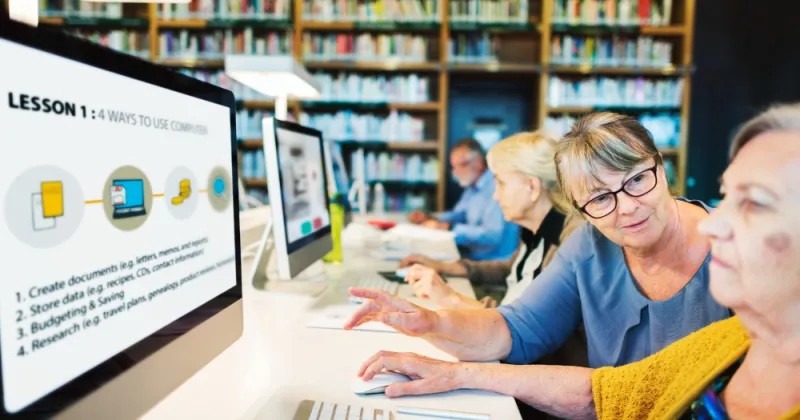
Evidence-Based AI-Enabled Teaching and Learning: A Critical Analysis
Introduction
AI is transforming education on all levels, including adult education, bringing innovative teaching methods that boost engagement and learning outcomes. Recent studies explore how Artificial Intelligence (AI) enhances project-based learning (PBL), inquiry-driven teaching, and evidence-based strategies using Large Language Models (LLMs). This analysis breaks down key findings, offering insights into the impact of AI on modern education and how it can be effectively integrated into adult educators' practices.
Theoretical Foundations of AI in Education
These studies emphasize the transformative potential of AI in education. Wan and Hu (2023) ground their research in constructivist theory—the idea that learners build knowledge by actively engaging with real-world problems. Their AI-powered project-based learning (PBL) model fits right into this framework, pushing learners to collaborate and tackle practical challenges together. Likewise, Xie (2023) explores AI’s role in inquiry-based teaching, showing how it helps learners sharpen critical thinking through questioning, gathering evidence, and problem-solving.
Mollick and Mollick (2023), on the other hand, take a slightly different angle. They lean on research from learning sciences and cognitive psychology to stress the importance of evidence-based teaching. They identify five teaching strategies that have been proven to boost learner learning—though, in practice, they’re not always easy to implement consistently. Hence, Mollick and Mollick (2023) lay a theoretical foundation for the use of Artificial Intelligence (AI), particularly Large Language Models (LLMs), as a tool to enhance and streamline the implementation of these evidence-based teaching strategies in educational settings.
Key Findings and Methodologies
Project-Based Learning and AI
Wan and Hu (2023) lay out a six-step model for integrating AI into project-based learning (PBL):
situational project design,
brainstorming,
role assignment,
activity exploration,
iterative optimization,
and achievement sharing.
Their approach is all about hands-on engagement with real-world tasks—learners don't just learn; they do. One example they give? Designing a vehicle-mounted device to optimize urban traffic using AI-enabled software. They argue that this method boosts creativity, teamwork, and engineering thinking. The key takeaway? AI learning works best when learners tackle real, meaningful projects they can complete within a short time frame: projects that demand they apply their knowledge in a way that makes sense in adult education.
Their contextual backdrop is the increasing importance of AI in basic education in China, driven by national development plans. The authors critically outline the specific steps involved in their proposed teaching mode, supporting their argument with pedagogical reasoning about stimulating creativity and improving practical abilities. While the paper describes the implementation steps and suggests positive outcomes, it lacks specific empirical data or formal evaluation to substantiate the claim of "good teaching results". The reliance on a single software example might limit the generalizability of their approach to other AI education contexts.
Inquiry-Based Teaching with AI
Xie (2023) takes a different route, focusing on how AI visualization technology, combined with advancements like 5G, can enrich teaching materials and create immersive learning experiences. The paper emphasizes the shift towards "intelligence" in education and the potential of AI to enhance teaching effectiveness and learner understanding. Inquiry-based teaching is at the heart of this discussion; an approach that nurtures learners' learning abilities and fosters active educator-learner interaction.
Regarding methodology, Xie (2023) uses a quantitative approach to measure the impact of AI-driven inquiry teaching. They break it down into four core elements—questioning, evidence acquisition, explanation focus, and evaluation summary—and, interestingly, both questioning and evaluation summary boost learning outcomes by 10%. Statistical tools like Cronbach’s α coefficient and a KMO value back up the study’s reliability. But even with these numbers, one has to wonder: how much of this applies outside the study’s specific setting?
The contextual backdrop includes the rapid development of AI in China and national policies promoting While this makes for an exciting research landscape, it also raises questions: how relevant are these findings in different educational systems or cultures? Moreover, Xie (2023) relies on self-reported data from questionnaires. As useful as these insights can be, there’s always the risk of bias, which could skew the study’s conclusions.
Implementing Effective Teaching Strategies with AI
Mollick and Mollick (2023) provide guidance on using AI, particularly Large Language Models (LLMs) like ChatGPT and Bing AI, to implement evidence-based teaching strategies that are often hard to execute due to time constraints. These strategies, each with its own theoretical basis drawn from educational research, form the core of their discussion:
Providing multiple and varied examples.
Offering multiple explanations.
Implementing frequent low-stakes testing.
Assessing learning.
Utilizing distributed practice.
For each, they provide practical guidelines and AI-generated prompts prompts to generate supporting material. However, they caution that educators must carefully evaluate AI’s output to ensure accuracy and relevance. Nevertheless, they argue that AI can act as a "force multiplier" for facilitators when used thoughtfully in service of evidence-based practices. The paper provides practical advice on evaluating and deploying AI-generated content in the educational setting.
Taking a pragmatic approach, Mollick and Mollick (2023) directly tackle the challenges educators face and present AI as a tool to help, not replace, educators The paper acknowledges the rapid adoption of LLMs in education and both the potential benefits and the inherent risks (e.g., AI hallucination). The authors urge educators to use AI critically, always verifying its accuracy before incorporating its output into their teaching.
The effectiveness of Mollick and Mollick’s (2023) approach ultimately depends on how well AI-generated content is vetted. They repeatedly stress that AI is a tool, not a replacement for educator expertise. Instructors must possess both subject matter and pedagogical knowledge to properly assess the AI’s contributions. Moreover, since the prompts provided are tailored to specific LLMs (like ChatGPT and Bing AI), future models might require adjustments.
Comparative Analysis
Strengths
These studies effectively demonstrate how AI can enhance educational practices:
Wan and Hu's PBL model fosters active participation through real-world projects.
Xie's inquiry-based approach systematically develops critical thinking and problem-solving skills.
Mollick and Mollick's work provides concrete ways for educators to implement evidence-based teaching strategies using AI, specifically Large Language Models (LLMs).
Limitations
Despite their strengths, these studies exhibit notable limitations:
Wan and Hu's qualitative findings lack empirical validation across diverse settings.
Xie's focus on Chinese higher education limits its applicability to other contexts.
Mollick and Mollick's paper emphasise a risk that AI-generated examples or explanations could be subtly wrong or could inadvertently reinforce learners' misconceptions if not carefully vetted by instructors.
None of the studies addresses potential challenges such as ethical concerns or disparities in access to AI technologies.
Implications for Practice
The findings from these studies have significant implications for educators. Incorporating real-world problems into training programs can make learning more relevant and engaging. Educators need training in using AI tools effectively to implement evidence-based teaching strategies and to facilitate inquiry-based or project-based learning. Policymakers should address issues like equitable access to AI technologies to ensure inclusive education.
Conclusion
AI-powered teaching methods are transforming adult education, potentially enhancing creativity, critical thinking, and real-world problem-solving skills. Wan and Hu (2023) highlight how project-based learning (PBL) nurtures innovation and collaboration, while Xie (2023) demonstrates how inquiry-based teaching can enhance learners' engagement and retention. Mollick and Mollick (2023) emphasize that AI, when applied strategically by educators, acts as a "force multiplier" to streamline evidence-based teaching strategies, improving learning efficiency and outcomes. Together, these studies lay a strong foundation for integrating AI into lifelong learning, though further research is needed to address challenges like AI accuracy, accessibility, ethics and instructor training.
References
Mollick, Ethan R. and Mollick, Lilach, Using AI to Implement Effective Teaching Strategies in Classrooms: Five Strategies, Including Prompts (March 17, 2023). The Wharton School Research Paper, Available at SSRN: https://ssrn.com/abstract=4391243 or http://dx.doi.org/10.2139/ssrn.4391243
Wan, C., & Hu, Z. (2023). Research on Application of Artificial Intelligence Teaching Mode Based on Project‐based Learning. International Journal of Emerging Horizons, 6(1), 121–122. https://doi.org/10.54097/ijeh.v6i1.3063
Xie, X. (2023). Influence of AI-driven Inquiry Teaching on Learning Outcomes. International Journal of Emerging Technologies in Learning, 18(23), 59–70. https://doi.org/10.3991/ijet.v18i23.45473
Comments
Hi @Georgi Timcenko Thank…
Thank you so much for your thoughtful engagement with my post and for highlighting such a critical gap between AI research and classroom reality—I couldn’t agree more. You’re absolutely right: understanding AI’s potential is one thing, but equipping educators with practical, hands-on skills to implement it ethically and effectively is another beast entirely.
Your mention of the AI Tools for Meaningful Learning and Training course resonates deeply. I’ve seen firsthand how "learning by doing" can demystify AI for educators. Structured training like this could be a game-changer for addressing the ethical dilemmas and accessibility hurdles you’ve outlined.
Let’s keep pushing for more collaborative spaces where theory meets practice.
Thanks again for sparking this conversation.
Best regards,
Elena Paspel
The blog post by Elena…
The blog post by Elena Paspel presents an insightful critical analysis of recent studies exploring the role of AI in education, particularly in adult learning. It does a great job of breaking down research on project-based learning, inquiry-driven teaching, and evidence-based instructional strategies, offering both praise and critique where necessary.
One of the most valuable aspects of this analysis is its focus on the practical applications and limitations of AI in education. The studies by Wan and Hu (2023), Xie (2023), and Mollick and Mollick (2023) all point to AI’s potential as a learning enhancer rather than a replacement for educators. The discussion about Large Language Models (LLMs) is particularly relevant today, as more educators explore how tools like ChatGPT can help streamline lesson planning, provide multiple examples, and enhance formative assessment.
Bridging Research and Practice: How Can Educators Apply These Insights?
While the post effectively highlights key findings, a crucial next step is considering how adult educators can develop the skills needed to integrate AI into their teaching practices. As pointed out in the limitations section, many studies focus on specific national contexts or theoretical applications without addressing the hands-on, real-world challenges educators face, such as ethical concerns, accessibility issues, and the risk of AI-generated misinformation.
To bridge the gap between theory and practice, educators need more than just knowledge of AI’s capabilities—they need direct experience using these tools in ways that align with their teaching goals. This is where practical, hands-on training can be invaluable.
A Practical Learning Opportunity: AI Tools for Meaningful Learning and Training
For educators who want to go beyond theoretical discussions and experience AI tools in action, the AI Tools for Meaningful Learning and Training course offers a structured, practice-based approach. This ERASMUS+ KA1 training course is designed for educators and training managers who want to develop their competencies in using AI tools to support self-directed, meaningful learning.
Participants will not only explore AI-driven teaching methods but also build a personal toolbox of AI tools, methods, and techniques applicable to their specific teaching contexts. One of the course’s key strengths is its learning-by-doing approach, where educators can test AI applications, reflect on their use from both a teacher and learner perspective, and develop strategies for ethical and effective AI integration.
For those inspired by the research discussed in Paspel’s blog post, this course provides an opportunity to translate those insights into practical teaching strategies. Given the rapid evolution of AI in education, having a hands-on experience can make a real difference in how educators navigate challenges, maximize benefits, and ensure AI truly enhances learning rather than complicating it.
For more details about the course, visit: AI Tools for Meaningful Learning and Training.